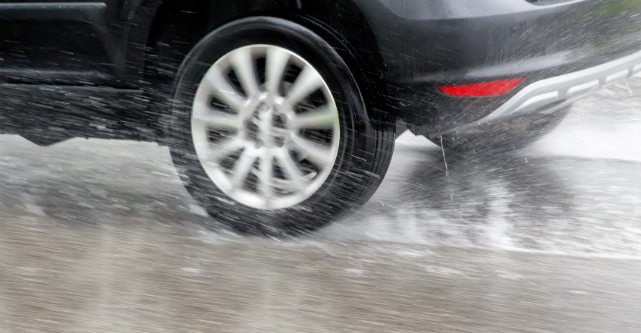
By Michael Milford
Would you rather a robot car that can drive you anywhere and at anytime, or a car that throws in the towel as soon as a storm hits or one that flat out refuses to take you anywhere at night?
No other challenge is bigger for the many Fortune 500 companies and startups battling to gain an edge in the self-driving car domain than developing an all-weather driverless car.
The billions of dollars invested in research and development to date has merely served as the prequel. We have many robot cars that can drive well “most of the time” but none that can drive well all of the time.
While it is tempting for companies to play up their success in fair weather, it’s hard to shy away from hard statistics: more than 20% of accidents in the United States are caused by adverse weather, for instance.
Looking further afield, if a company is to gain traction with billions of potential customers across Asia, it must create cars that can confidently carry you through even the worst monsoonal storm.
It’s not just rain either; snow, ice, sleet, fog, smoke, dust, wind, glare and heat can all play havoc with driving conditions.
Humans do it better, for now
We humans manage to drive in these conditions, mostly accident free, because of how well we are able to perceive and interpret the world around us, even in the middle of a snowstorm.
We can do amazing things, like infer that the tiny bit of red sticking out from the top of a snowbank is probably a stop sign, and act accordingly. We can see a clear reflection of another car in a puddle of water and slow down but not stop, knowing that what we’re seeing is a reflection, not the real thing, but also that we should slow down as we drive through the water.
The two dominant approaches to self-driving cars lie at opposite ends of the spectrum. The first is the “brute force” approach, as employed by companies such as Google, which has relied heavily on extensive reconnaissance of the street network beforehand, and an army of humans labelling these maps with all the useful practical information required to drive sensibly along those roads.
The second approach takes in thousands or millions of kilometres of driving data from car-mounted radar, lasers and cameras as well as the car’s control interface, and learns “how to drive” using algorithmic learning methods.
The recent resurgence of deep learning – multi-layered neural networks that are trained with vast quantities of data – has played a central role in these learning-based approaches.
The approaches, as well as hybrid combinations of both, have gotten us to the stage where a car can drive itself using an array of often expensive sensors mounted around the car and on the roof, only making occasional mistakes every few hours that require human intervention.
At least in fine weather. But at night, or in heavy rainfall, snow or fog, most of these systems are useless.
The ‘blind’ driverless car
Some of the blame has been attributed to the sensors themselves. Many lasers don’t see well through heavy rain, and cameras don’t work as well in low light conditions at night. In these conditions, the car is effectively blind.
But this cannot be the only reason. The next time you’re a passenger in a car driving in a tropical thunderstorm or snowstorm at night, think about what the human driver can actually see.
Odds are, they can’t see more than a few dozen metres in front of the car. Between windscreen wiper sweeps, rain or snow falling from the sky and kicked up by the car in front will drastically impede visibility.
Oncoming headlights will regularly blind the driver, and the lane markings will be nearly impossible to distinguish. Distant objects such as pedestrians will disappear into the rain and gloom.
Unlike robot cars that use roof-mounted lasers to calculate centimetre-accurate range readings to every single thing around the car up to 120 metres away in all directions, a human driver relies primarily on their eyes and intuition.
It is our visual intelligence that enables us to drive in these conditions without veering off the road or crashing into cars and pedestrians that we can barely see.
The fact that a human driver can drive under conditions that make any current robot car grind to a halt is both incredibly daunting and tantalising for companies and researchers working on self-driving cars.
We have the ultimate proof of concept that it can be done – ourselves – but not yet the deep understanding of how we do it.
The first company to achieve this understanding and then build it into their self-driving cars will be optimally placed to win this trillion dollar race and ensure our robot cars are always there for us, especially when we need them the most.
Michael Milford is an associate professor at Queensland University of Technology.
This article was originally published on The Conversation. Read the original article.
Follow StartupSmart on Facebook, Twitter, LinkedIn and Soundcloud.
COMMENTS
SmartCompany is committed to hosting lively discussions. Help us keep the conversation useful, interesting and welcoming. We aim to publish comments quickly in the interest of promoting robust conversation, but we’re a small team and we deploy filters to protect against legal risk. Occasionally your comment may be held up while it is being reviewed, but we’re working as fast as we can to keep the conversation rolling.
The SmartCompany comment section is members-only content. Please subscribe to leave a comment.
The SmartCompany comment section is members-only content. Please login to leave a comment.