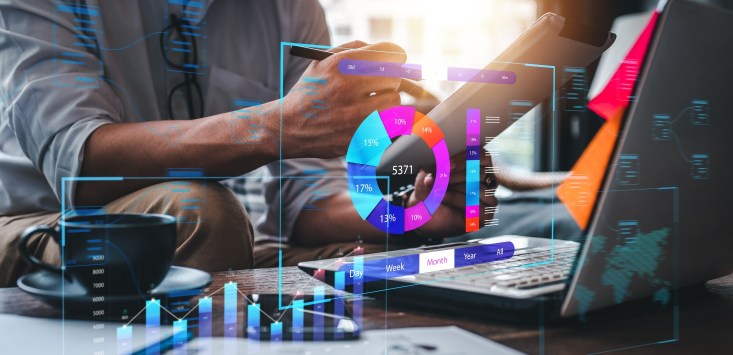
Source: Adobe Stock
The postcard view of artificial intelligence, or AI, in business is to sail into the sunset, free of time-consuming tasks as you soak up the benefits of data-driven insights.
Data scrubbing is not in that postcard.
Cleaning or scrubbing your data sounds like a thankless chore – and in some ways, it is – but it’s a crucial step to ensure your AI ship doesn’t sink long before it gets to your burden-free paradise.
Dirty data is information that is invalid, unchecked, or captured incorrectly, and it can damage the health and accuracy of your database, which is costly in terms of inaccurate forecasts and missed opportunities.
As many as 85% of businesses are affected by poor data quality, in wasted resources and additional costs (40%), damaged reliability of analytics (36%) and negative effects on customer relationships and trust (32%), according to data analytics firm Experian.
Poor data hygiene can also lead to serious compliance problems, such as violating data privacy laws, which can easily happen if documents are fed ad hoc into AI tools, exposing sensitive information such as names, bank details and personal records.
Why you need to clean your data
So why is it so important?
Data hygiene underpins the architecture used for AI. You can’t ask a machine to learn from a data set and expect it to ignore material that is incorrect, out of date, poorly described, biased, or only telling a partial story.
It becomes critical, then, for businesses to clean up their data by identifying errors or inconsistencies, removing personal or sensitive information that shouldn’t be used in the training set, and organising data for consistency and reliability.
Take, for example, an analysis of your sales. If most sales take place face-to-face but a portion are made online, and your two systems barely speak, then any analysis will produce a skewed view of the products you sell or customer behaviour.
If some of your suppliers send paper invoices that are simply paid, while others deliver e-invoices in a system able to capture multiple fields, the same challenge applies.
Until now this has been a problem only once a business has chosen to adopt AI — a deliberate decision that usually includes other levels of system transformation.
But almost every common ERP and work platform provider is now rushing to add in AI elements, meaning an abrupt upgrade of payroll, invoicing, time and attendance, sales, marketing and customer management software, as well as ordinary tools like Microsoft Word, Outlook and Excel.
What we find is that many businesses run a combination of technologies. They might invoice on a modern system like Xero, but they will also have legacy systems – enterprise resource planning software that is 20 years old, a dozen point solutions that are not connected, or paper-based resources that are the definition of dirty data.
Focus on the ‘why’ of using AI
How can a business with an untidy legacy of paper trails and incomplete data sets start its AI journey?
The first step is to understand the business case for AI, by focusing on the opportunity or problem that you are trying to solve, rather than starting with the platform.
Is the user case really clear to the business? It must tell a story about what it will deliver for the business and why it is worth investing the time.
The second element is understanding what data will be needed to address that business case and in what state of cleanliness it is, and to start the scrubbing process.
Not every document created by a company will be necessary for the model, and there is a need to avoid low-value material such as out-of-date or conflicting documents.
The next step is to consider where you are going to work and the appropriate tools, which means considering the privacy implications as well as the fitness of the tool for the purpose.
As hyped AI tools are rushed to market and promising to change the world, there can be a temptation to act fast but it is important not to rush in blindly and consider strategy.
Take the time to consider marginal gains that might come with using bespoke data to increase the training material and body of work used to drive the AI, versus the immediate application of off-the-shelf models being rolled out.
Stay focused on the problem or opportunity where the biggest gains are going to be made.
Finally, many of the common challenges around AI arise from operational landscapes where legacy software has outlived its usefulness.
This might be the moment to rethink your tech architecture and take steps to clean up the legacy-based mess while looking at the opportunities from the latest innovations.
The postcard AI utopia can be achieved, but there’s some work to be done first. Getting your house in order means you can sail into the AI sunset without sinking the ship.
Sudha Viswanathan is the director of analytics and insights at Pitcher Partners.
Handpicked for you
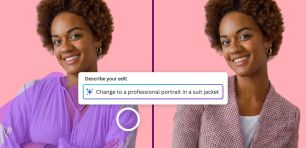
Canva just mic-dropped 10 new AI tools, and they don’t cost extra
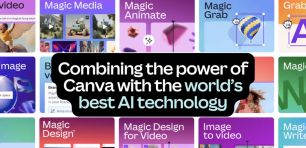
COMMENTS
SmartCompany is committed to hosting lively discussions. Help us keep the conversation useful, interesting and welcoming. We aim to publish comments quickly in the interest of promoting robust conversation, but we’re a small team and we deploy filters to protect against legal risk. Occasionally your comment may be held up while it is being reviewed, but we’re working as fast as we can to keep the conversation rolling.
The SmartCompany comment section is members-only content. Please subscribe to leave a comment.
The SmartCompany comment section is members-only content. Please login to leave a comment.